Manufacturing is one of those sectors that moved with digital technologies at a snail’s pace. However, with statistics, it is proved that this sector is definitely realizing the importance and the benefits AI brings forth with numerous AI in manufacturing examples.
A survey showed that 89% of international manufacturers will deploy AI in production networks soon and that 68% have already started deploying AI. However, only 16% of manufacturing firms have achieved their goals due to several issues related to a lack of digital skills and scalability. Nevertheless, AI and digital technology, combined with employees’ higher skill levels, have been and will continue to create a major boost for this industry.
Curious about how applications of AI in manufacturing are changing this field? Below, we have rounded up some of the most actionable examples of AI use cases in manufacturing, helping businesses improve their efficiency in operations.
7 AI in Manufacturing Examples
AI in manufacturing examples plays a pivotal role in bringing about an essential alteration. AI helps the manufacturing industry get improvement and efficiency that is unparalleled. Here are 7 AI use cases in manufacturing.
1. Cobots & autonomous mobile robots
Cobots are a popular application of AI in manufacturing. Cobots or collaborative robots form a very integral part of the production cycle in that they help in increasing productivity by working alongside operators. Commonly, fulfillment centers deploy robots that pick up and pack various products. These robots work in conjunction with workers, allowing them to move around complex areas and find objects with AI-powered technology.
According to the “9th Annual State of Smart Manufacturing” report by Rockwell Automation, many manufacturers are now using cobots and autonomous robots to enhance and support their workforce, reduce errors, accelerate value creation, and improve quality. A survey shows that around 85% of manufacturing businesses have committed or are considering investment in AI or machine learning this year.
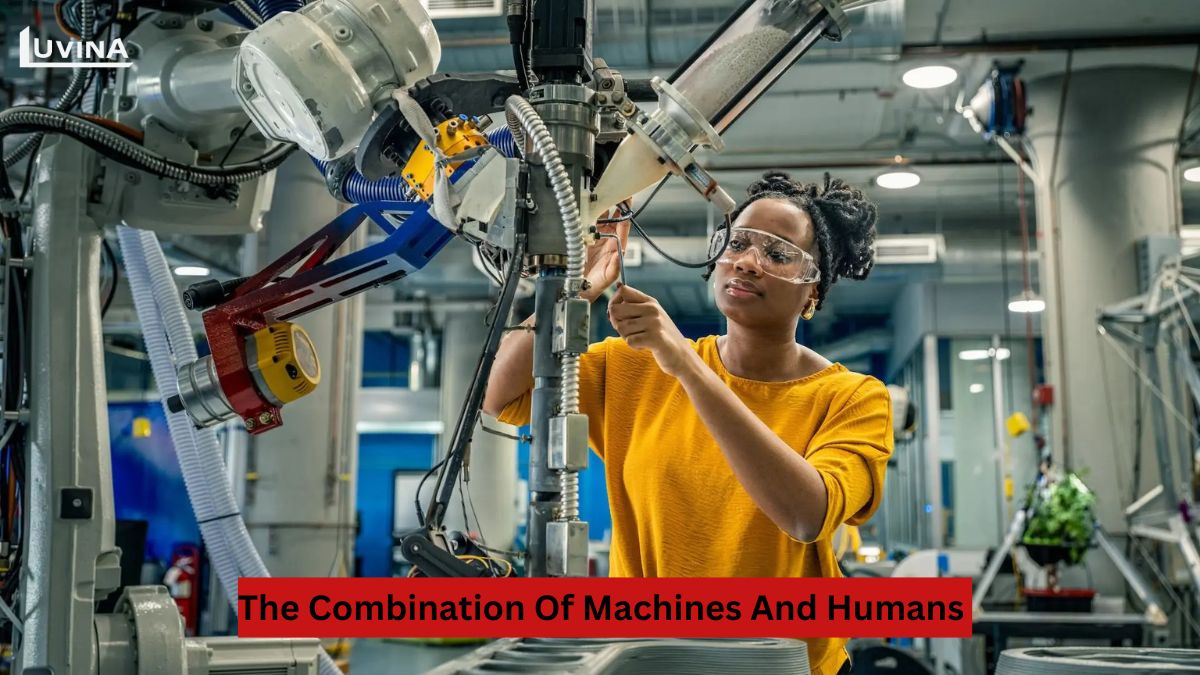
More examples of AI in manufacturing use cases that are directly involved with cobots or autonomous robots will be seen. For example, Amazon uses machine learning to accelerate the order fulfillment process and to smoothen operations and logistics. Already, this e-commerce giant has deployed over 750,000 robots into action, working alongside employees and performing complicated tasks with uncanny precision and agility. These cobots can conduct complex assembly processes, and quality control, and work together with human employees seamlessly.
More precisely, Amazon has just introduced a new robot tool called Sequoia, which identifies and stores the inventory in fulfillment centers 75% faster than current competitors. With this use of artificial intelligence in industry, Amazon has reduced order processing time by 25%, minimized downtime, lowered maintenance costs, and ensured optimal efficiency.
Amazon’s use of AI in manufacturing is a great example of how cobots and AI can work together to create a fast and productive manufacturing environment where machines and humans cooperate harmoniously to achieve excellence in all operations.
Apart from Amazon, Ford also stands out among AI in manufacturing examples. The company has installed robotic arms that pick up metal converters and assemble them into position. While performing these tasks, the AI system learns the most efficient ways to assemble the remaining components.
According to a survey by ABI Research, drones are also capturing the interest of many companies in the manufacturing field.
2. Quality Control
Every error in the production process affects the quality of the final product. That’s why many artificial intelligence applications in manufacturing focus on quality control. By using AI in manufacturing, companies can reimagine the process of quality control by guaranteeing superior precision and consistency. AI has been able to demonstrate such exceptional accuracy in quality control that is just unbeatable by humans.
Typically, AI-powered computer vision systems analyze images or sensor data to detect defects. Machine learning algorithms are trained on labeled data to recognize patterns associated with defects, enabling automated defect classification and sorting.
Furthermore, the use of AI in the manufacturing industry extends to predictive quality assurance. By analyzing historical and real-time sensor data, machine learning algorithms can detect patterns and trends that signal potential quality issues. This allows manufacturers to proactively address potential defects and implement corrective actions before they impact the final product.
One of the leading names in AI applications for manufacturing quality control is the electronics manufacturer Foxconn. To improve its quality control processes, Foxconn has integrated AI technology and computer vision, where AI systems quickly and efficiently detect electronic component defects by examining images and videos, ensuring products meet rigorous standards. With AI, Foxconn enhances production efficiency and accuracy, producing high-quality goods on a large scale in the electronics field.
Among other AI in manufacturing examples, BMW stands out with its own AI platform, AIQX (Artificial Intelligence Quality Next). This is a quality control platform that uses sensor camera technology and AI to automate quality inspections on the production line. AI records data in real time and sends immediate feedback to workers on the production line through smart devices.
3. Performance Optimization
In manufacturing, performance optimization is a crucial aspect, and the use of AI in the industry is a true game-changer. Specifically, AI algorithms are used to identify patterns, detect anomalies, and make data-driven predictions—all capabilities that allow manufacturers to streamline operations, minimize downtime, and improve the overall effectiveness of equipment.
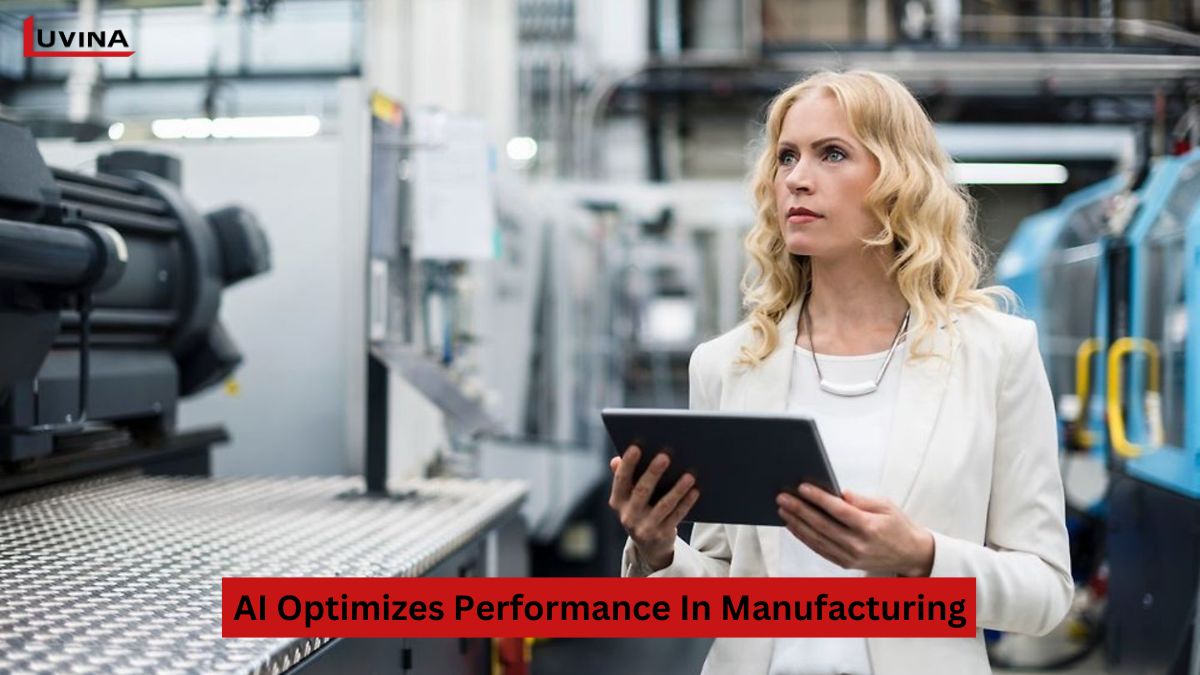
Examples of AI in manufacturing that help transform processes and optimize factory performance include General Electric (GE). GE has integrated AI algorithms into its production processes to analyze vast amounts of data from historical records and sensor devices. With AI’s support, GE has been able to identify trends to predict equipment-related issues and optimize workflows. This proactive approach has enabled GE to minimize equipment downtime, increase overall equipment efficiency, and ultimately maximize operational effectiveness in production.
4. Forecasting demand to optimize the supply chain
Machine learning in manufacturing examples not only helps distributors manage supply chains more effectively but also prepares them for future disruptions.
Artificial intelligence in manufacturing industry examples for demand forecasting brings numerous benefits. It allows manufacturing companies to make data-driven decisions based on analyses of sales data, sales history, market trends, and external factors. As a result, manufacturers can anticipate demand fluctuations and adjust production processes accordingly, minimizing risks of stockouts or excessive inventory.
There are many AI in manufacturing examples for demand forecasting. For instance, a fashion manufacturer could use AI to predict demand for clothing items by analyzing data from various sources like weather forecasts, customer preferences, and social media trends. The AI system can provide accurate forecasts, enabling the fashion retailer to optimize inventory levels and ensure popular items are always available.
5. Predictive maintenance
Although it is not the primary application, predictive maintenance would also be one of the most popular and game-changing uses of AI ML use cases in manufacturing as far as improving the production process goes. Using advanced analytics, machine learning, and prediction algorithms, AI solutions for manufacturing allow companies to actively equip monitoring, and predict failures, reducing downtime while optimizing scheduling. AI analyzes data collected from remote sensors and other sources for patterns predictive of when equipment is likely to fail.
Relating to AI in manufacturing case studies for predictive maintenance, some key concepts to note are digital twins and generative AI. A digital twin is a virtual copy of a physical asset that can gather data in real time and simulate the virtual behavior of the physical asset. When a digital twin is hooked up with sensor data, AI analyzes patterns and anomalies, and a prediction of imminent failure is determined. This in turn helps maintenance teams to predict and undertake proactive repair work before the breakdown occurs.
One of the best manufacturing AI use cases for predictive maintenance is Ford’s use of digital twin technology. Ford creates different digital twins for each model it produces, with each twin handling a separate area—from concept to operation. Ford also made digital models for the manufacturing process, production facilities, and even customer experience. The digital twin of production facilities at Ford can accurately highlight the energy losses and indicate the areas where energy saving can improve the entire efficiency of the production line.
Another important name when discussing AI use cases in the manufacturing industry is Rolls-Royce. It has developed a digital twin platform to consolidate data from its produced aviation engines to maintain them more optimally. It enables Rolls-Royce to monitor their performance, predict any likely failures, and also optimize maintenance schedules based on analysis of real-time and historical data from these engines. By integrating digital twins and AI, Rolls-Royce has been able to deliver operational efficiency, safety, and reliability within the aviation industry.
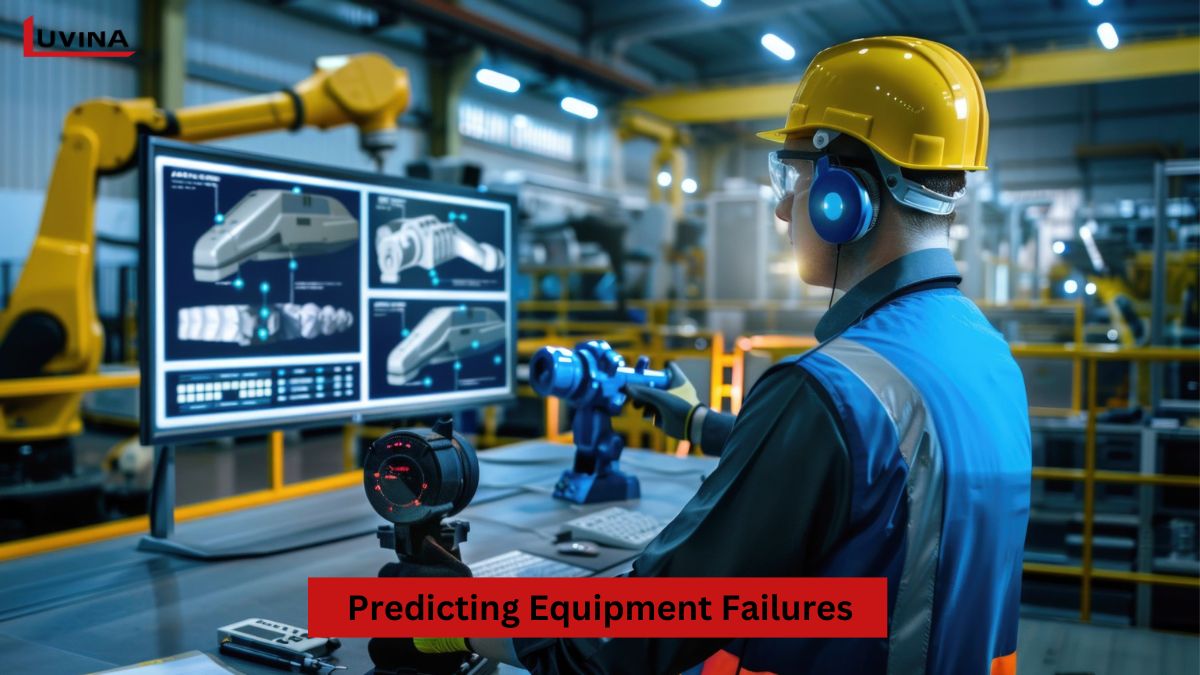
Another concept among use cases of AI in manufacturing related to predictive maintenance is generative AI. Generative AI, a subset of deep learning, learns from existing datasets to create new content, such as text, images, or code. In manufacturing, generative AI can create synthetic data to simulate potential failure scenarios. This synthetic data can then be used to train predictive maintenance models.
In the manufacturing field, companies understand that with a robust data analysis solution, they can determine when machines will stop working, identify root causes, and gain deeper insights to effectively resolve issues and avoid business disruptions. That is why predictive maintenance is expected to remain one of the leading industrial AI use cases in the coming years.
6. Managing inventory
Currently, AI in manufacturing examples is not limited to production processes but has expanded to include inventory management both before and after production. AI systems can also evaluate historical data, detect constraints in the production line, and adjust inventory levels accordingly.
Specifically, AI can:
- Monitor inventory levels and material flow.
- Analyze historical sales data, current inventory levels, and market trends to accurately predict demand.
- Alert when inventory is low.
Manufacturing machine learning use cases allows warehouses to optimize inventory levels, reduce storage costs, and ensure product availability.
7. Autonomous vehicles
List any four use cases of AI in manufacturing, and among them, autonomous vehicles will be included. Autonomous vehicles are currently one of the most prominent uses and applications of artificial intelligence in manufacturing, although the deployment of autonomous vehicles in manufacturing is still at various stages and remains relatively new.
However, the uses of AI in manufacturing for autonomous vehicles have been integrated by many companies, especially in the automotive manufacturing sector. One of the most notable examples is the automaker Rivian – which has integrated AI predictive technology into the R1T pickup truck and the R1S SUV and is now rolling out initiatives to integrate traditional AI and genAI into the vehicles.
Furthermore, Tesla has announced plans to install a $500 million Dojo supercomputer at its Gigafactory in New York to train its AI system for autonomous driving. The Dojo supercomputer will process millions of terabytes of data from Tesla electric vehicles to improve the safety and technical performance of the autonomous driving features that Tesla provides.
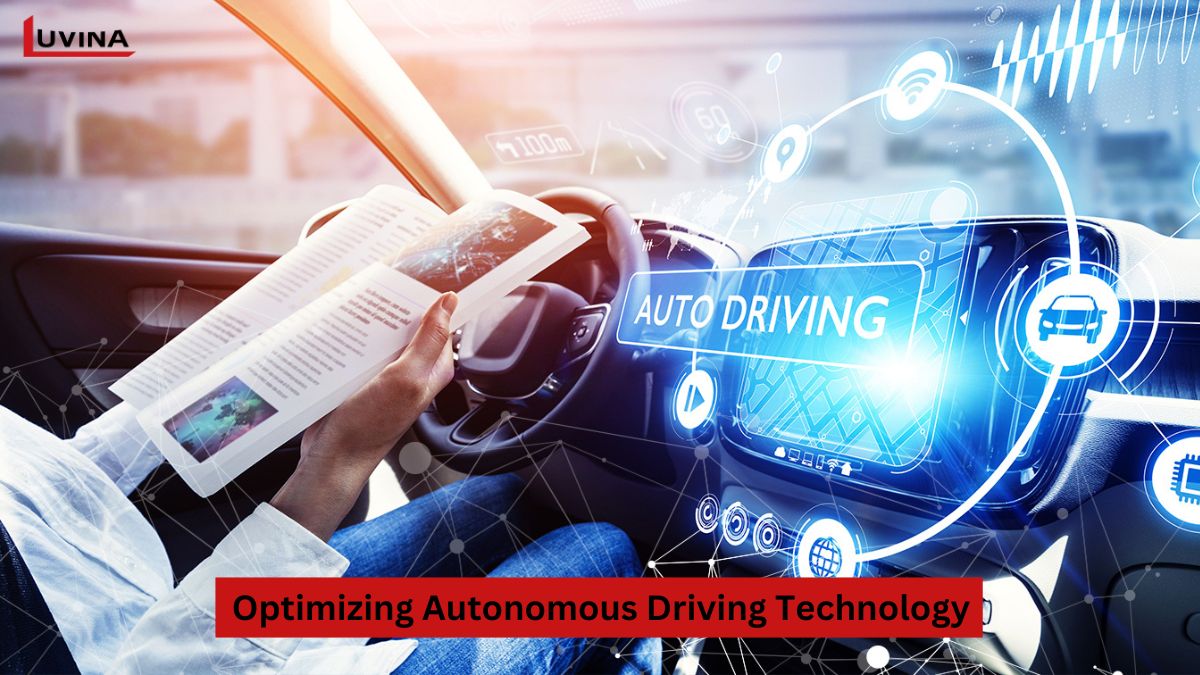
Another typical example of AI for manufacturing companies is the British automaker Bentley. Bentley is currently focusing on plans to deploy a level 2 autonomous system, taking over control functions such as remote parking assistance, driving, and speed management. However, this technology from Bentley is still in the development phase because it still requires the driver to remain focused on the road.
Conclusion
AI use cases in manufacturing, such as quality control, inventory management, and predictive maintenance, have proven effective in helping businesses improve performance, increase safety, and enhance decision-making. At the same time, there are many challenges businesses may face, such as issues related to the digital gap or data infrastructure. However, it cannot be denied that the future of the manufacturing industry will definitely have a place for AI.
I hope the article above has provided you with valuable insights into the 7 applications of AI in manufacturing. Although AI in manufacturing is still in its early stages at this moment, as the ROI from AI tools becomes clearer and the technology continues to improve, AI in manufacturing examples will become more diverse.
Related Posts:
Read More From Us?
Sign up for our newsletter
Read More From Us?
Sign up for our newsletter